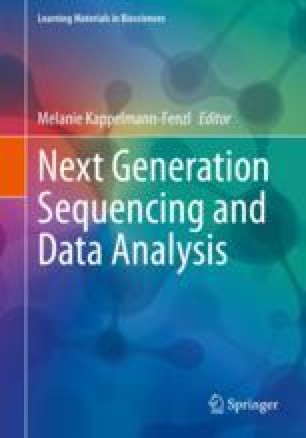
New Book Chapter: Design and Analysis of RNA Sequencing Data
In this chapter, we introduce the concept of RNA-Seq analyses. First, we start to provide an overview of a typical RNA-Seq experiment that includes extraction of sample RNA, enrichment, and cDNA library preparation. Next, we review tools for quality control and data pre-processing followed by a standard workflow to perform RNA-Seq analyses. For this purpose, we discuss two common RNA-Seq strategies, that is a reference-based alignment and a de novo assembly approach. We learn how to do basic downstream analyses of RNA-Seq data, including quantification of expressed genes, differential gene expression (DE) between different groups as well as functional gene analysis. Eventually, we provide a best-practice example for a reference-based RNA-Seq analysis from beginning to end, including all necessary tools and steps on GitHub: https://github.com/grimmlab/BookChapter-RNA-Seq-Analyses.