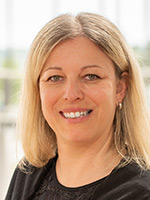
Jasmin joins the team as Team Assistant
We welcome our new Team Assistant Jasmin in our team.
We welcome our new Team Assistant Jasmin in our team.
Ashima joins the team as research assistant. She will work on novel machine learning methods for synthetic protein design and the in silico evaluation of generated artificial sequences.
Our latest paper on horticultural demand forecasting has been published in Expert Systems with Applications: “Forecasting seasonally fluctuating sales of perishable products in the horticultural industry”. Accurately forecasting demand is a potential competitive advantage, especially in the context of perishable products such as in the horticultural industry, where the disposal of unsold items results in environmental and financial damage. Despite challenging operational decisions to avoid out-of-stock and overstock situations, horticultural businesses have received limited attention in forecasting research. In addition, horticultural sales are typically highly seasonal. Sudden changes in both directions, rising and falling, characterize horticultural sales cycles.In our study, we explore the research questions of the applicability of general versus dataset-specific predictors, the impact of external information, and online model update schemes. Using a diverse set of real-world horticultural data, we applied three classical and twelve machine learning-based forecasting approaches.💡 Key Findings:Multivariate machine learning models dominate: Our results show the superiority of multivariate machine learning methods over classical forecasting approaches, with the ensemble learner XGBoost emerging as a standout performer.External factors play a critical role: The inclusion of statistical, calendrical, and weather-related features in the feature set is critical for robust performance.Firm-specific predictors outperform general cross-firm models: We find that a generalized model, which would be advantageous in terms of computational resources, maintenance, and transferability to other datasets, falls short in capturing the heterogeneity of horticultural data, highlighting the need for firm-specific predictors.Impact of frequent model updates is negligible: Surprisingly, frequent model updates have a negligible impact on forecast quality, allowing long-term forecasting without significant performance degradation.
New paper about an impressive manually annotated and curated dataset of diverse weed species in maize and sorghum for computer vision. Here we present a dataset, the Moving Fields Weed Dataset (MFWD), which captures the growth of 28 weed species commonly found in sorghum and maize fields in Germany. A total of 94,321 images were acquired in a fully automated, high-throughput phenotyping facility to track over 5,000 individual plants at high spatial and temporal resolution. A rich set of manually curated ground truth information is also provided, which can be used not only for plant species classification, object detection and instance segmentation tasks, but also for multiple object tracking.
New paper about a Protein Language model-based Thermophilicity predictor (ProLaTherm). ProLaTherm significantly outperforms all feature-, sequence- and literature-based comparison partners on multiple evaluation metrics.