News
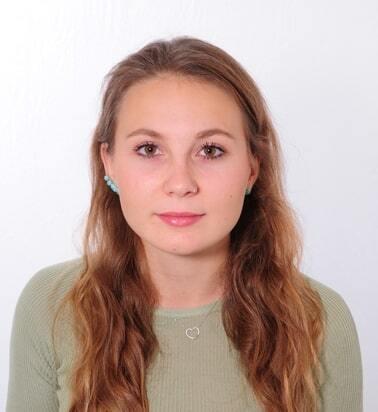
Sofia joins the Team as Research Assistant
Sofia joins the team as research assistant. She will work on novel machine learning methods for synthetic protein design and the in silico evaluation of generated artificial sequences.
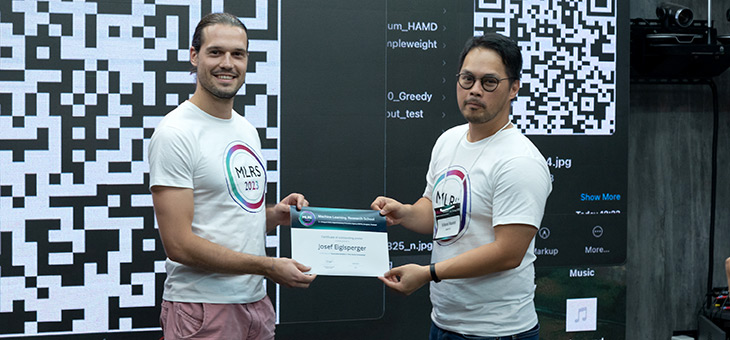
Machine Learning Research School in Bangkok: Poster Award für Josef Eiglsperger
Award for Josef Eiglsperger: The doctoral student at the Professorship of Bioinformatics (Prof. Dominik Grimm) at Weihenstephan-Triesdorf University of Applied Sciences (HSWT) at TUM Campus Straubing (TUMCS) received the “Outstanding Poster Award” for his poster and presentation at the Machine Learning Research School (MLRS) in Bangkok. The Research Summer School ran from 02.08. to 09.08.2023 and took place at the Digital Economy Promotion Agency.
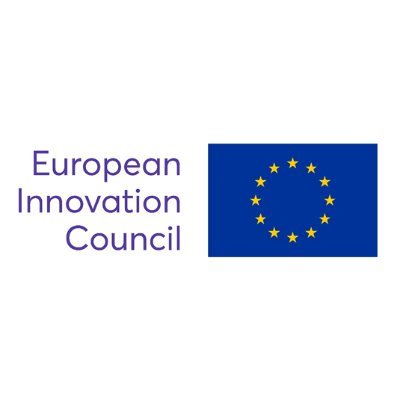
First EIC Transition Funding for TUM
We are delighted to have received funding from the European Innovation Council (EIC) as part of the EIC Transition programme to work with colleagues at the Technical University of Munich (TUM) to develop a new technology that will make it quicker, easier and more accurate to apply fertiliser in the field. Using a combination of biosensor test strips and satellite-based remote sensing data, the Technical University of Munich (TUM) is developing a method to determine the nutritional status of cereals and the perfect amount of fertiliser to apply. The automatic provision of digital analysis data to the tractor terminal should save time and prevent over-fertilisation in the future. The Straubing Campus for Sustainability plays a leading role within the TUM in the development of environmentally friendly technologies. The GrimmLab will be responsible for the development of the machine learning related parts for fertilizer recommendations.
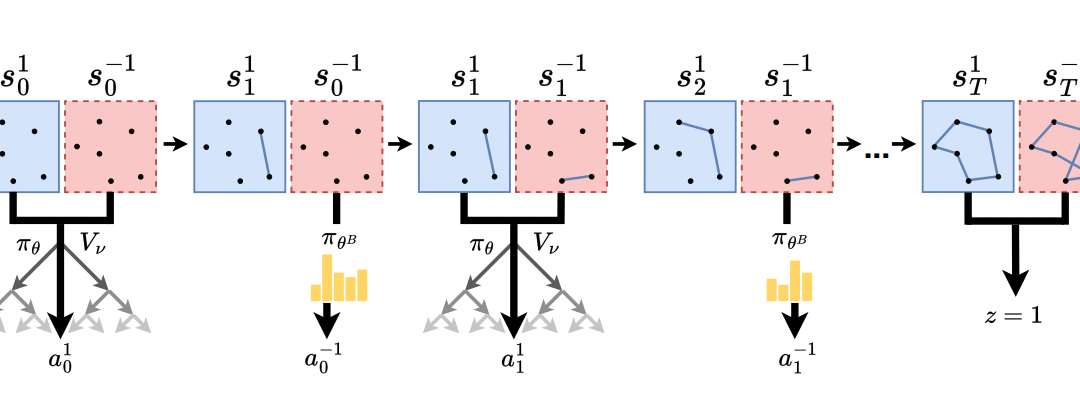
New Paper: Policy-Based Self-Competition for Planning Problems
New paper at International Conference on Learning Representation (ICLR): “Policy-Based Self-Competition for Planning Problems”. AlphaZero-type algorithms may stop improving on single-player tasks in case the value network guiding the tree search is unable to approximate the outcome of an episode sufficiently well. One technique to address this problem is transforming the single-player task through self-competition. The main idea is to compute a scalar baseline from the agent’s historical performances and to reshape an episode’s reward into a binary output, indicating whether the baseline has been exceeded or not. However, this baseline only carries limited information for the agent about strategies how to improve. We leverage the idea of self-competition and directly incorporate a historical policy into the planning process instead of its scalar performance. Based on the recently introduced Gumbel AlphaZero (GAZ), we propose our algorithm GAZ ‘Play-to-Plan’ (GAZ PTP), in which the agent learns to find strong trajectories by planning against possible strategies of its past self. We show the effectiveness of our approach in two well-known combinatorial optimization problems, the Traveling Salesman Problem and the Job-Shop Scheduling Problem. With only half of the simulation budget for search, GAZ PTP consistently outperforms all selected single-player variants of GAZ.
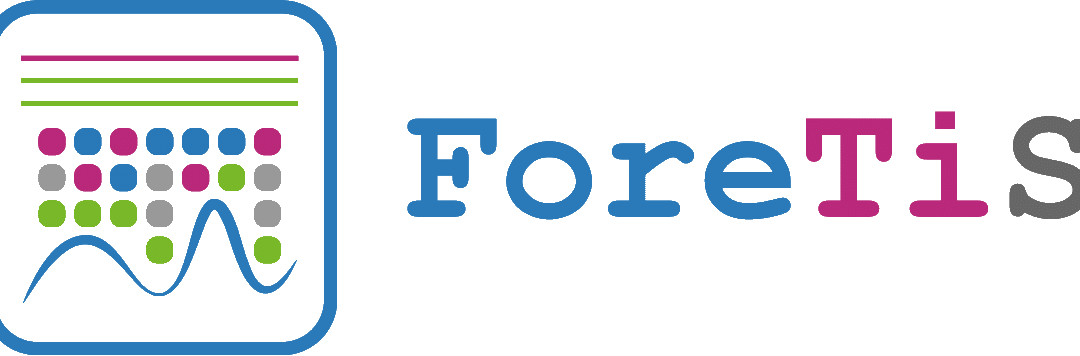
New Paper: ForeTiS – a comprehensive time series forecasting framework in Python
New paper in Machine Learning with Applications: “ForeTiS: A comprehensive time series forecasting framework in Python”. Time series forecasting is a research area with applications in various domains, nevertheless without yielding a predominant method so far. We present ForeTiS, a comprehensive and open source Python framework that allows rigorous training, comparison, and analysis of state-of-the-art time series forecasting approaches. Our framework includes fully automated yet configurable data preprocessing and feature engineering. In addition, we use advanced Bayesian optimization for automatic hyperparameter search. ForeTiS is easy to use, even for non-programmers, requiring only a single line of code to apply state-of-the-art time series forecasting. Various prediction models, ranging from classical forecasting approaches to machine learning techniques and deep learning architectures, are already integrated. More importantly, as a key benefit for researchers aiming to develop new forecasting models, ForeTiS is designed to allow for rapid integration and fair benchmarking in a reliable framework. Thus, we provide a powerful framework for both end users and forecasting experts.
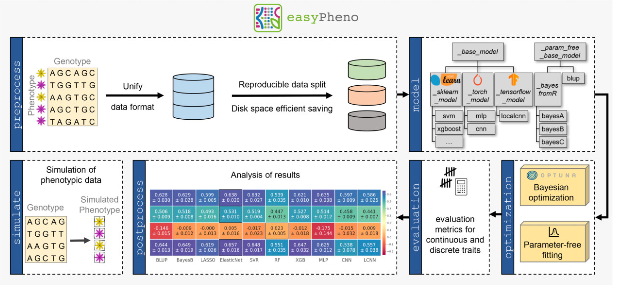
New Paper: easyPheno, an easy-to-use and easy-to-extend Python framework for phenotype prediction using Bayesian optimization
New paper in Bioinformatics Advances: “easyPheno: An easy-to-use and easy-to-extend Python framework for phenotype prediction using Bayesian optimization”. Predicting complex traits from genotypic information is a major challenge in various biological domains. With easyPheno, we present a comprehensive Python framework enabling the rigorous training, comparison and analysis of phenotype predictions for a variety of different models, ranging from common genomic selection approaches over classical machine learning and modern deep learning-based techniques. Our framework is easy-to-use, also for non-programming-experts, and includes an automatic hyperparameter search using state-of-the-art Bayesian optimization. Moreover, easyPheno provides various benefits for bioinformaticians developing new prediction models. easyPheno enables to quickly integrate novel models and functionalities in a reliable framework and to benchmark against various integrated prediction models in a comparable setup. In addition, the framework allows the assessment of newly developed prediction models under pre-defined settings using simulated data. We provide a detailed documentation with various hands-on tutorials and videos explaining the usage of easyPheno to novice users.
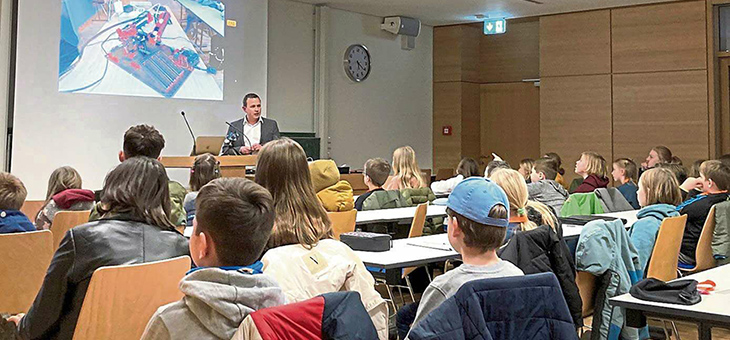
Ist ein Computer schlau?
„Ist ein Computer dumm oder schlau, was meint ihr?“ Mit dieser Frage hat Prof. Dr. Grimm, Leiter der Professur für Bioinformatik, die erste Vorlesung der Kinderuni im Jahr 2023 eröffnet. Mithilfe von Lego- Steinen und eines Roboterarms machten sich die jungen Forscher mit Prof. Grimm auf die Suche nach einer Antwort.
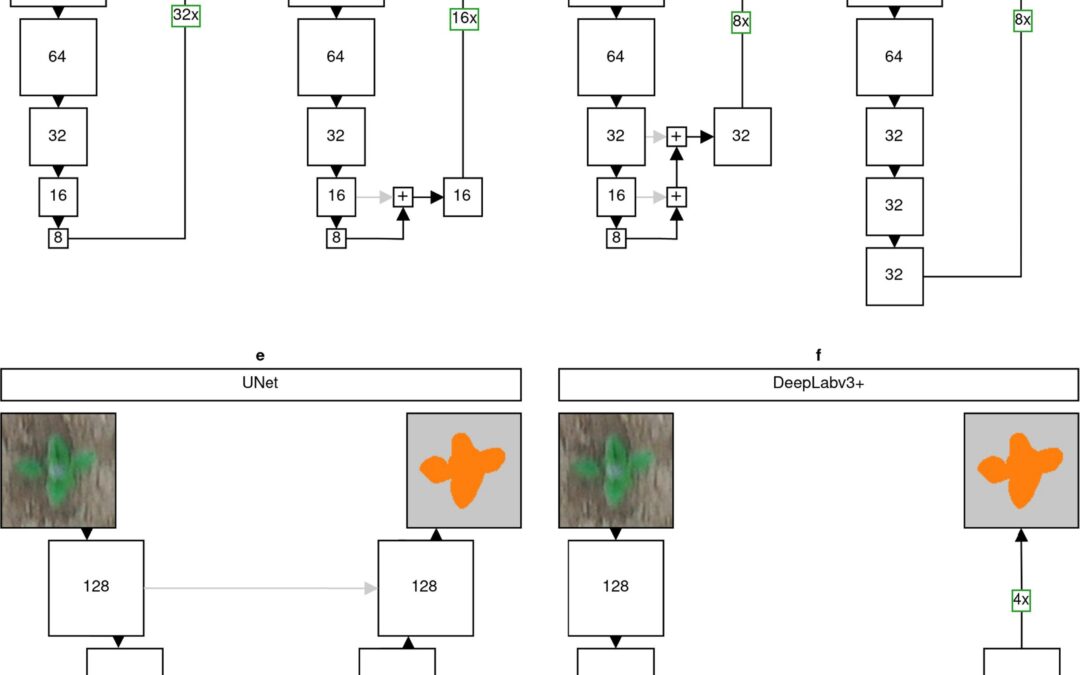
New StMELF Funding: AI for Weed Regulation with Robots
We are happy to receive funding from the Bavarian State Ministry of Food, Agriculture and Forestry to continue or research about developing novel machine learning techniques for weed identification in drone imagery to enable automatic weed removal due to autonomous robots on agricultural fields.
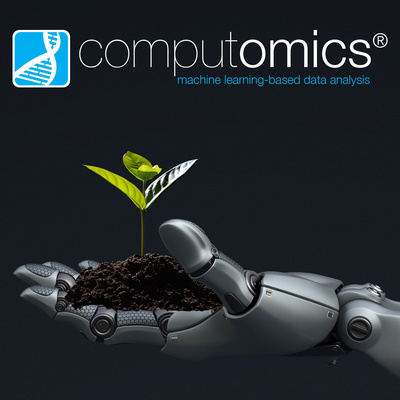
Dominik talks at the Computomics Podcast about ML for agriculture
In this episode Dominik gives us insights into CropML, a BMBF funded project. The project evaluates new machine learning techniques for more accurate plant breeding by integrating heterogeneous external factors. Different phenotype prediction models, including basic genomic selection methods to more advanced deep learning-based techniques have been compared. Learn why advanced models are the future and where the challenges are.
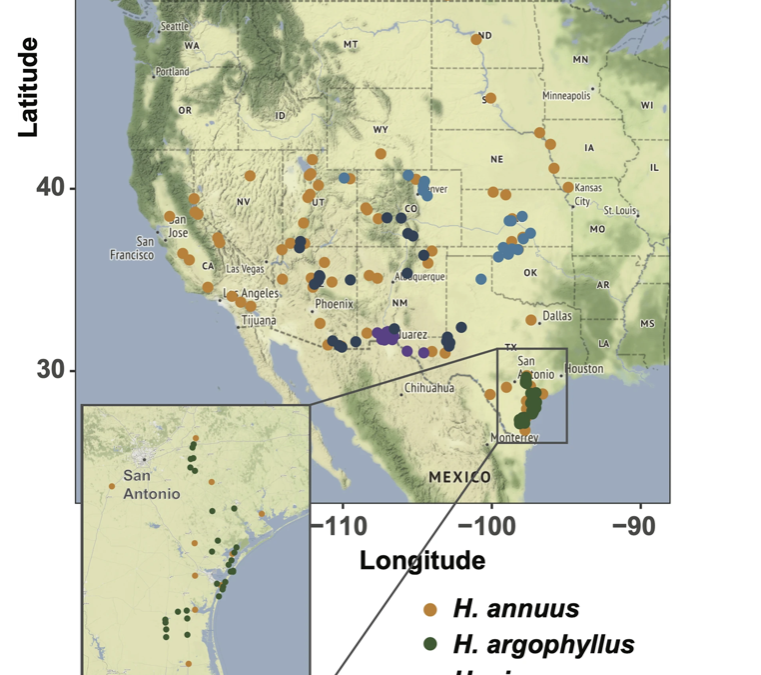
New Paper: HeliantHOME, a public and centralized database of phenotypic sunflower data
New paper in Scientific Data: “HeliantHOME, a public and centralized database of phenotypic sunflower data”. We have created HeliantHOME (http://www.helianthome.org), a curated, public, and interactive database of phenotypes including developmental, structural and environmental ones, obtained from a large collection of both wild and cultivated sunflower individuals.