News
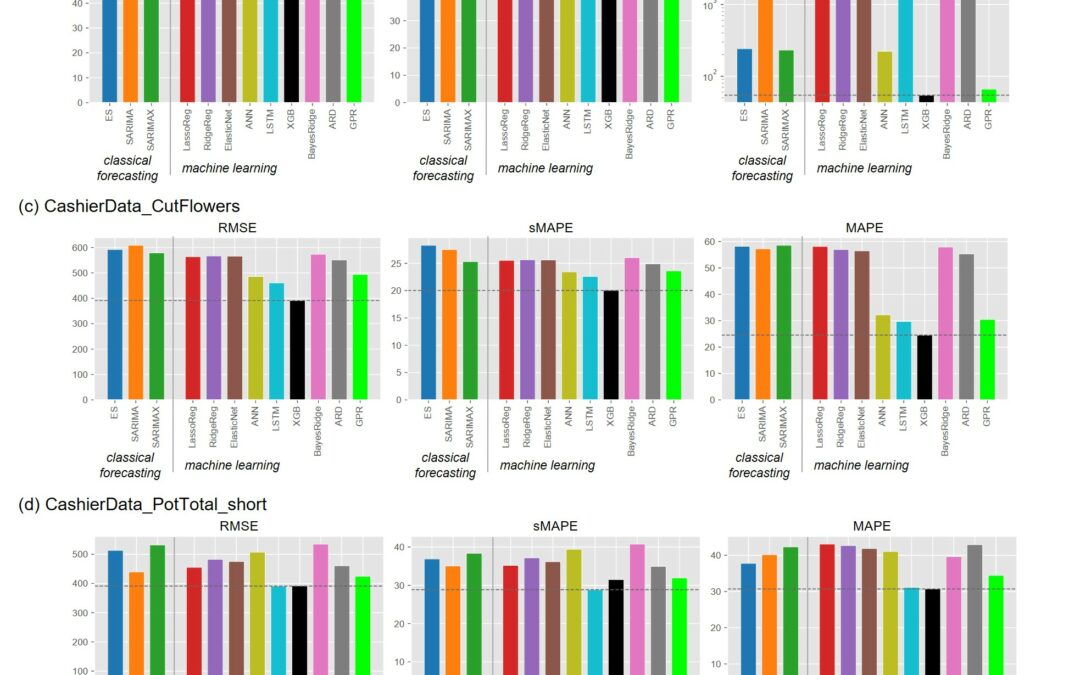
New Journal Paper about Machine Learning for Horticultural Sales Predictions
We published an original research paper on a comparative study with several Machine Learning and classical forecasting algorithms to predict horticultural sales.
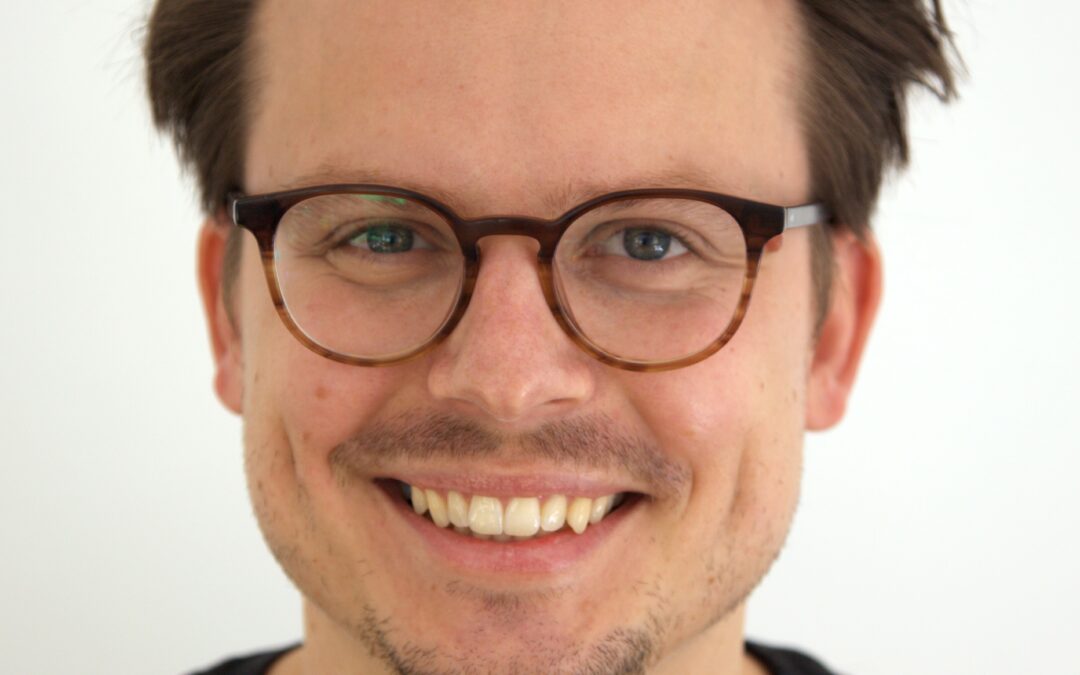
Jonathan joins the Team as PhD Student
Jonathan joins the team as PhD students. He will work on novel machine learning and reinforcement learning problems within the project “Reinforcement Learning for Automated Flowsheet Synthesis of Steady-State Processes”, funded by the German Research Foundation (DFG).
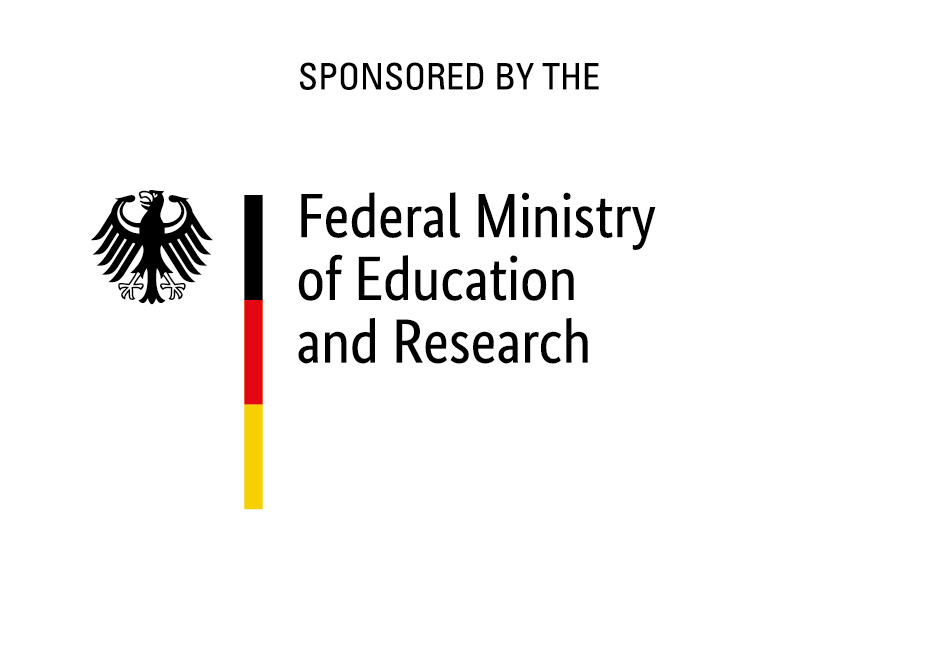
New BMBF Funding
Together with Computomics GmbH we successfully attracted funding from the Federal Ministry of Education and Research (BMBF) for our new project “CropML: New machine learning techniques for more accurate plant breeding by integrating heterogeneous external factors”.
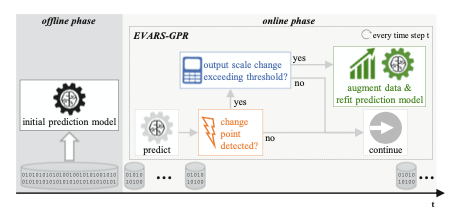
New Paper and Conference Talk about EVARS-GPR
We published a novel machine learning algorithm EVARS-GPR an EVent-triggered Augmented Refitting of Gaussian Process Regression for Seasonal Data. Florian presented the method at the German AI Conference. The talk can be found on youtube: https://youtu.be/jZ6hZNMa-TE
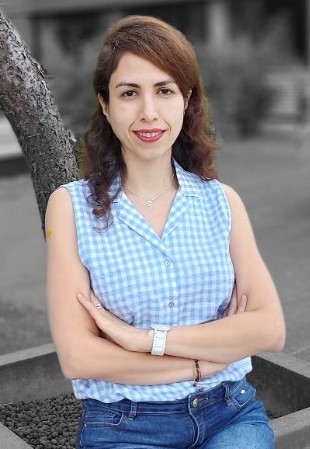
Sara joins the Team as PostDoc
Sara will advance and support the further development of the Synthetic Biology Foundry.
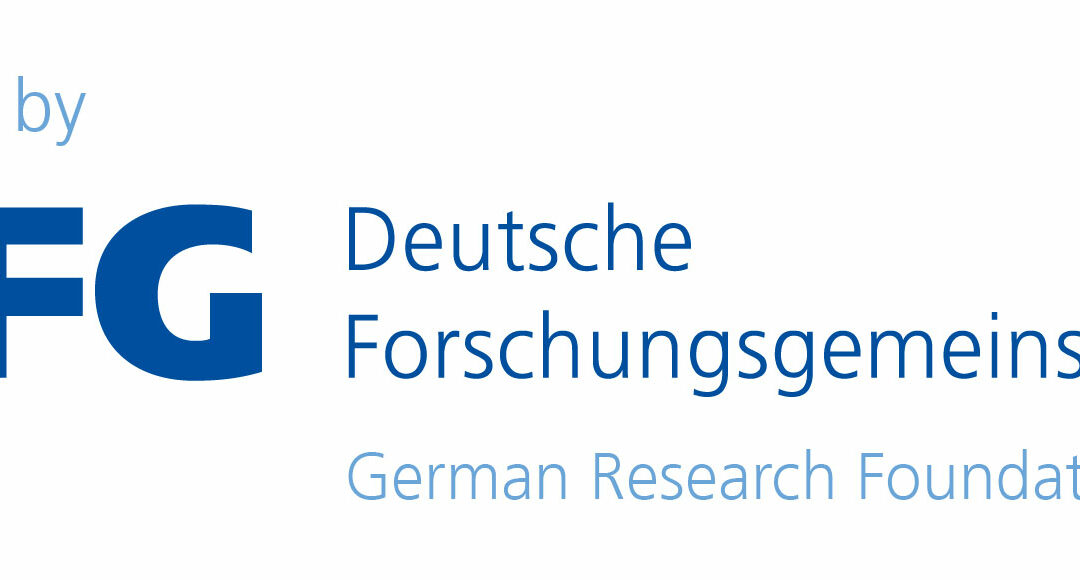
New DFG Funding
We successfully attracted funding from the German Research Foundation (DFG) for the project “Reinforcement Learning for Automated Flowsheet Synthesis of Steady-State Processes”
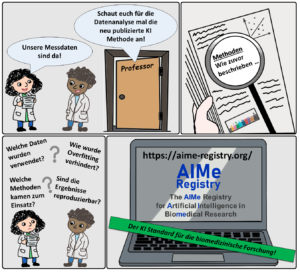
New Publication in Nature Methods: The AIMe registry for artificial intelligence in biomedical research
An international research team with participants from several universities including Prof. Dr. Dominik Grimm has proposed a standardized registry for artificial intelligence (AI) work in biomedicine to improve the reproducibility of results and create trust in the use of AI algorithms in biomedical research and, in the future, in everyday clinical practice. The scientists presented their proposal in the scientific journal “Nature Methods”.
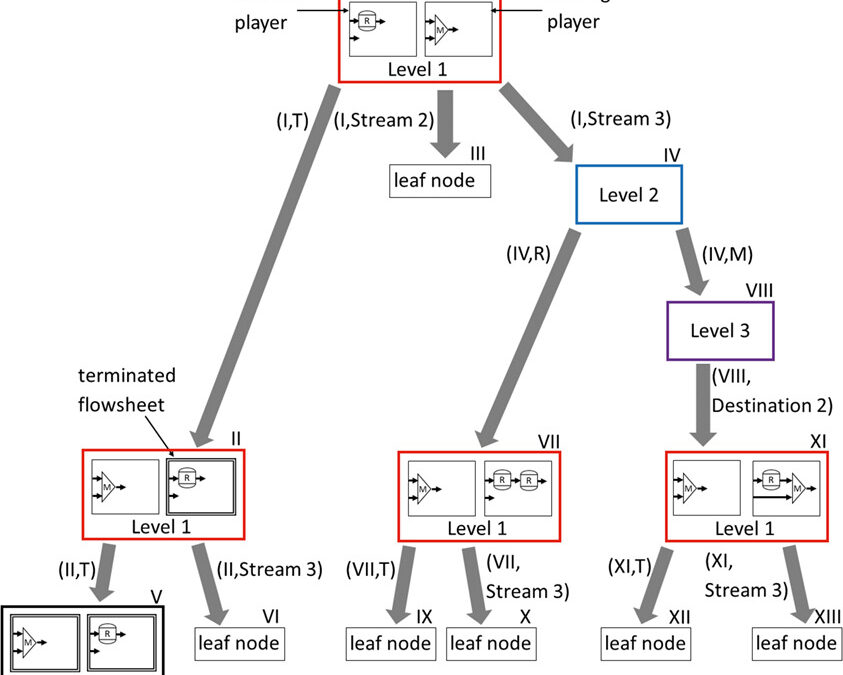
New Publication: Automated Flowsheet Synthesis Using Hierarchical Reinforcement Learning
Recently we showed that reinforcement learning can be used to automatically generate process flowsheets without heuristics or prior knowledge. For this purpose, SynGameZero, a novel two-player game has been developed. In this work we extend SynGameZero by structuring the agent’s actions in several hierarchy levels, which improves the approach in terms of scalability and allows the consideration of more sophisticated flowsheet problems. We successfully demonstrate the usability of our novel framework for the fully automated synthesis of an ethyl tert-butyl ether process.
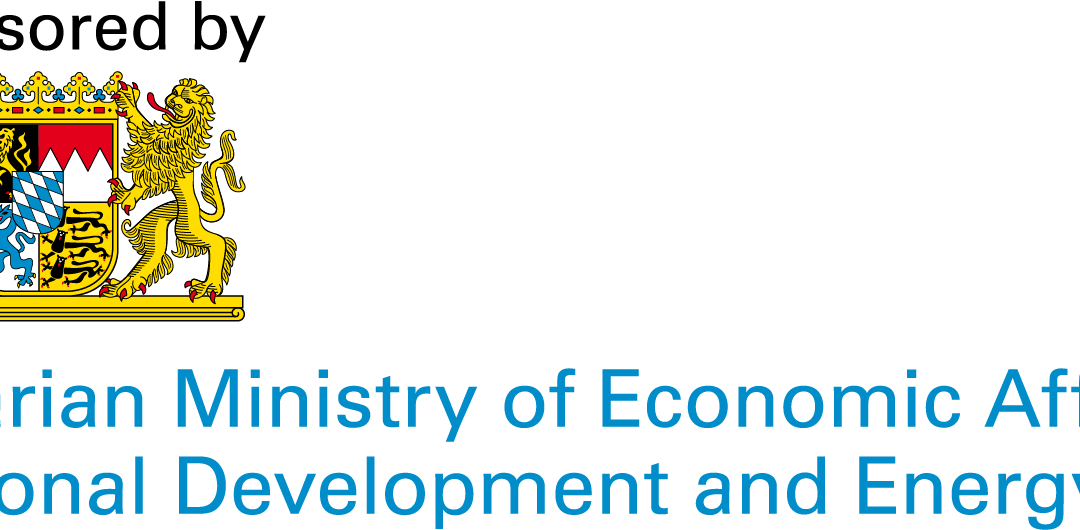
New Funding: Glycoside Production
We successfully attracted funding for a new project from the Bavarian Ministry of Economic Affairs, Regional Development and Energy. More details will follow soon on our project page.
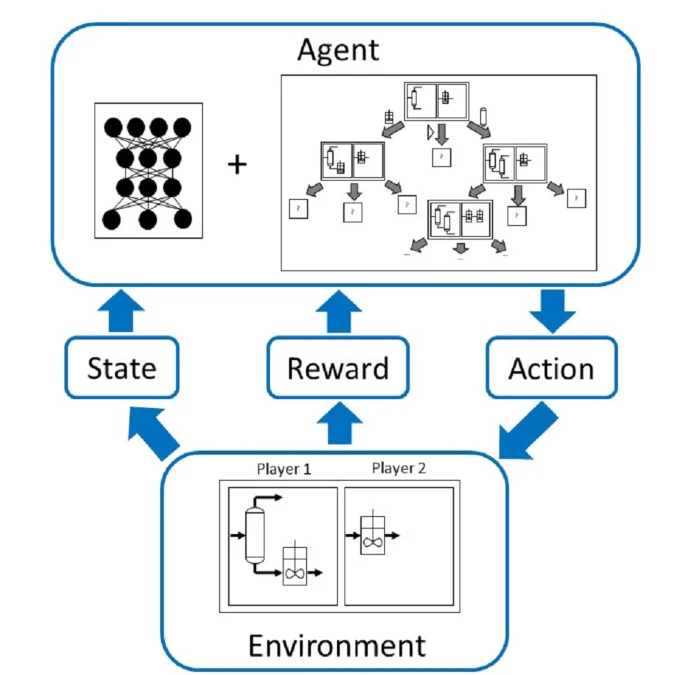
New Publication: Automated Process Synthesis Using Reinforcement Learning @ ESCAPE31
A novel method for automated flowsheet synthesis based on reinforcement learning (RL) is presented. Using the interaction with a process simulator as the learning environment, an agent is trained to solve the task of synthesizing process flowsheets without any heuristics or prior knowledge. The developed RL method models the task as a competitive two-player game that the agent plays against itself during training. The concept is proven to work along an example with a quaternary mixture that is processed using a reactor or distillation units.