We are collecting and publishing several data resources as part of our research. Subsequently, you can find a brief description of our datasets and references for further information. If you are interested in more details and discussions, do not hesitate to contact us.
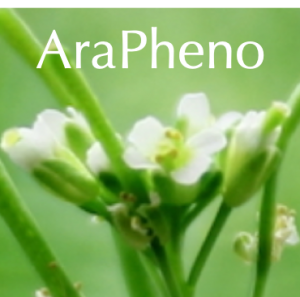
AraPheno is a central and public repository of high-quality Arabidopsis thaliana phenotypes. This database allows to search and filter for public phenotypes and to obtain additional meta-information. For more information, see the two publications given here.
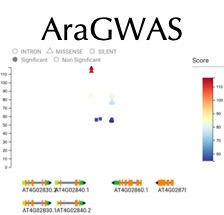
The AraGWAS Catalog is a public and manually curated database for standardised GWAS results for Arabidopsis thaliana. This database allows to search and filter for public GWASs, phenotypes and genes and to obtain additional meta-information. All GWASs were recomputed following a uniformed methodology to allow for comparable results. For more information, see this publication.
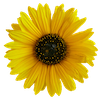
HeliantHOME is a a public and centralized database home of a comprehensive collection of phenotypes for different sunflower species. We include developmental, structural, and environmental phenotypes, obtained from a large collection of both wild and cultivated sunflower individuals. Additionally, the database is enriched with external genomic data and results of GWASs. For more information, see this publication.
ProtThermoPred is a benchmark dataset for protein thermophilicity prediction consisting of significantly updated datasets from literature and newly collected data. Besides FASTA files containing protein sequences, we provide meta information related to the proteins. For more information, see the description in our GitHub repository.
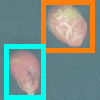
Seed Germination contains captures of germination experiments of 824 Zea mays, 811 Secale cereale, and 814 Pennisetum glaucum seeds. Approximately ten seeds were placed in one petri dish and captured by a Raspberry Pi Camera Module (v2.1) in intervals of 30 minutes for about two days. All images were annotated with bounding boxes containing their germination state. For more information, see this publication.
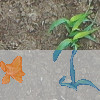
UAV Weed Segmentation is a manually-annotated and expert-curated drone image dataset for weed detection in sorghum fields in Southern Germany at growth stage BBCH 17. During image capture, several weed species were present on the field. A consumer-grade drone was used at a flight altitude of five meters above ground level with a corresponding ground sampling distance of one millimeter. For more information, see this publication.
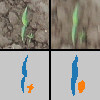
Deblurring Weed Segmentation is a manually-annotated and expert-curated drone image dataset for weed detection in sorghum fields (growth stage BBCH 13) consisting of image-pairs with and without motion blur. UAV captures were generated at an experimental agricultural sorghum field in Southern Germany, and deblurred using a deep learning-based model. For more information, see our GitHub repository.
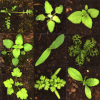
The Moving Fields Weed Dataset is a manually annotated and curated dataset of diverse weed species in maize and sorghum for computer vision. It consists of 640 plots of 28 weed species (monocots and dicots), 6 varieties of Sorghum and 2 varieties of Maize. Each plot consists of a timeseries of images resulting in > 82.000 images in total. Plenty ground-truth annotations are provided as well, ranging from bounding boxes over time-series tracks to semantic and instance segmentation labels for selected plots. For more information, see here.